Welcome to a groundbreaking exploration of the Best AI Breakthrough that promises to revolutionize the world of Artificial Intelligence. In this blog post, we will delve into the cutting-edge techniques that are set to unlock limitless Explainability, paving the way for unprecedented transparency and understanding in AI systems. Prepare to be amazed as we reveal the jaw-dropping Number 6 – a game-changer that will leave you in awe!
1) What is Limited Explainability in AI?
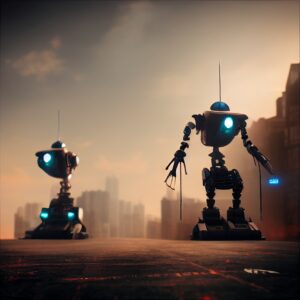
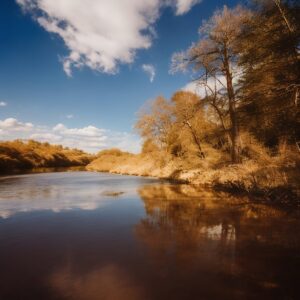
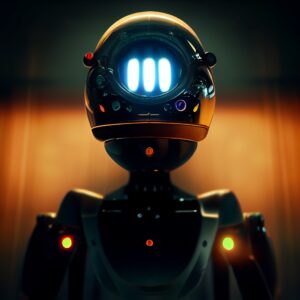
Before we dive into the solutions, let’s understand the concept of limited Explainability in AI. Currently, many AI models operate as black boxes, making it difficult for researchers, developers, and even end-users to comprehend their decision-making processes. This lack of transparency raises concerns about accountability, trust, and the potential for biased outcomes.
2) The Quest for Explainable AI
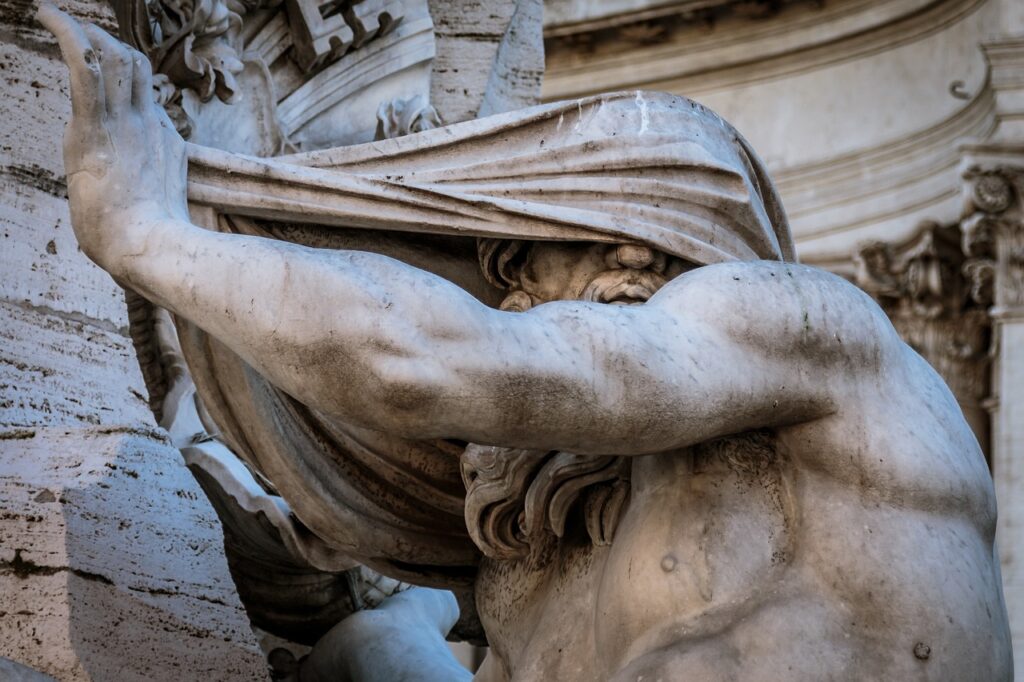
In recent years, the pursuit of explainable AI has intensified, with researchers and organizations striving to make AI systems more interpretable without sacrificing performance.
Here are some of the most promising breakthroughs that are reshaping the AI landscape:
2.1 Interpretable Deep Learning Architectures
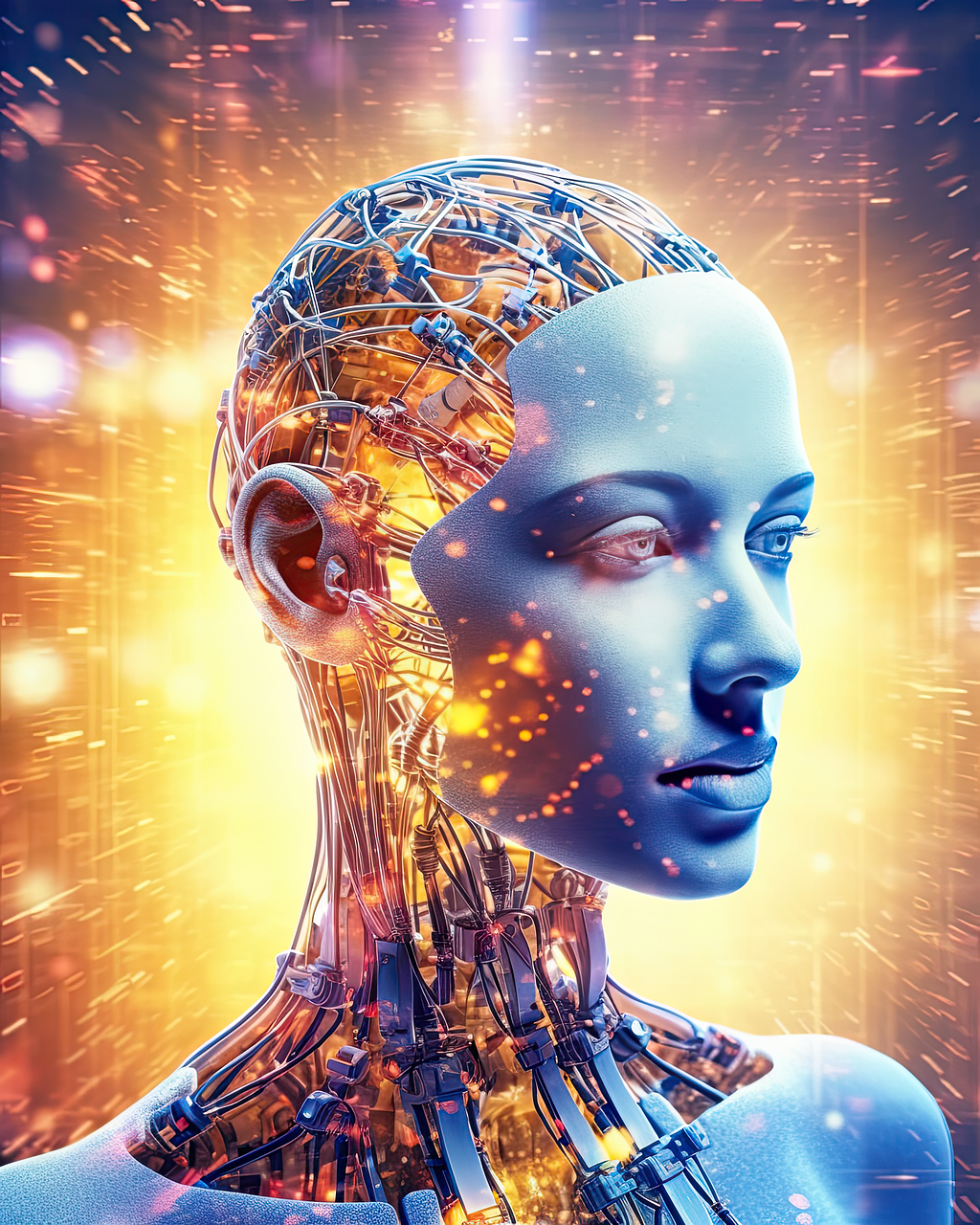
Pros of Interpretable Deep Learning Architectures: Improved transparency, easy validation/debugging.
Cons of Interpretable Deep Learning Architectures: Reduced complexity, limited applicability for complex tasks.
Researchers have developed novel deep learning architectures that provide insights into how AI models arrive at their conclusions. Techniques like attention mechanisms and gradient-weighted class activation mapping (Grad-CAM) offer visualizations that shed light on the critical features influencing the model’s decisions.
2.2 Rule-based Systems

Pros of Rule-based Systems: Clear decision-making process, easy to understand and interpret.
Cons of Rule-based Systems: Limited complexity, may not handle nuanced or ambiguous situations well.
Rule-based AI systems are designed to work on if-then principles, where the reasoning behind each decision can be traced back to specific rules. This approach allows for transparency and easy validation of AI outputs.
2.3 LIME (Local Interpretable Model-agnostic Explanations)
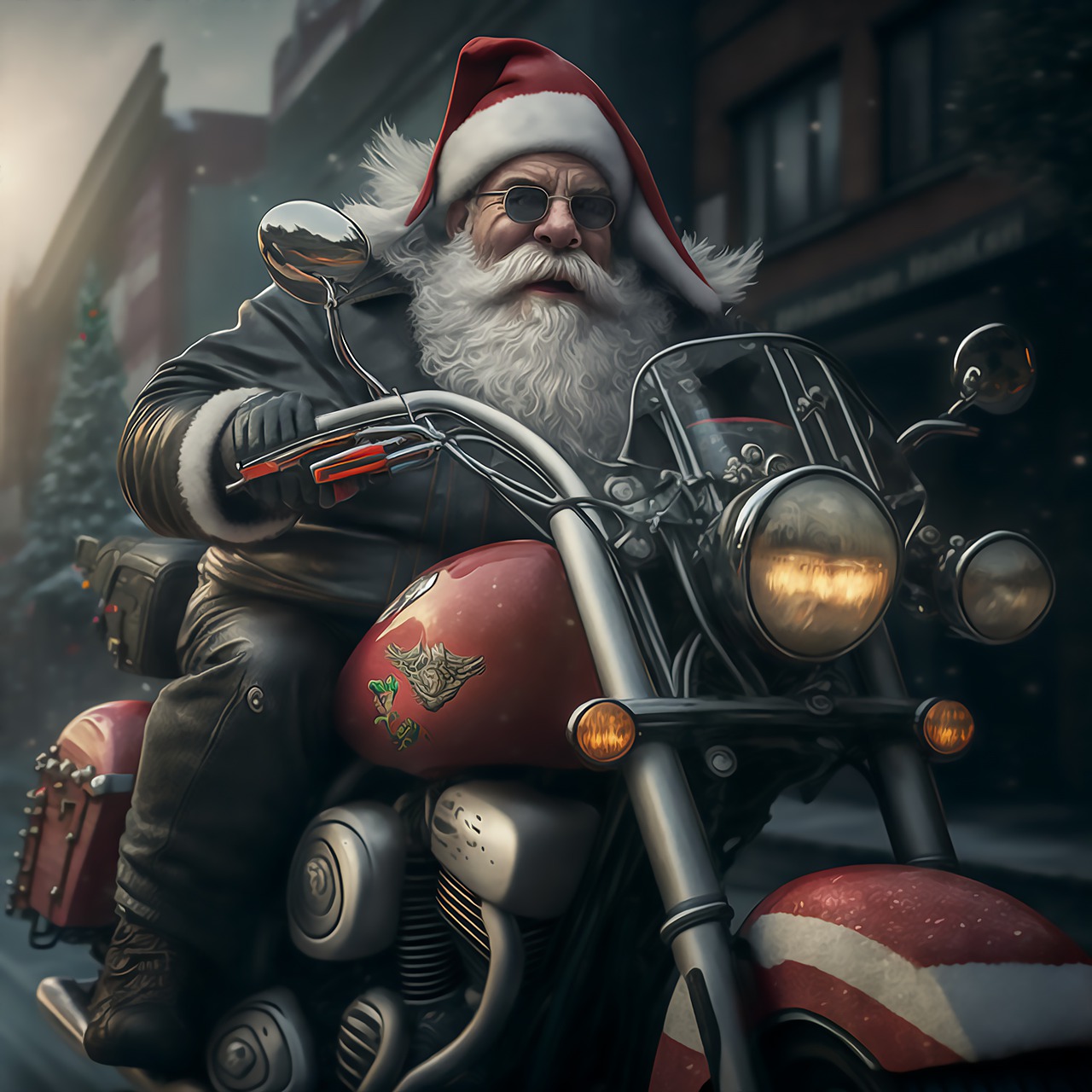
Pros of LIME : Model-agnostic, provides interpretable explanations for complex models.
Cons of LIME: Limited to local interpretations, may not capture global model behavior accurately.
LIME is a groundbreaking technique that helps explain the predictions of complex AI models by approximating them with simple, interpretable models. This allows us to gain insights into the model’s decision process at a local level.
2.4 SHAP (SHapley Additive exPlanations)
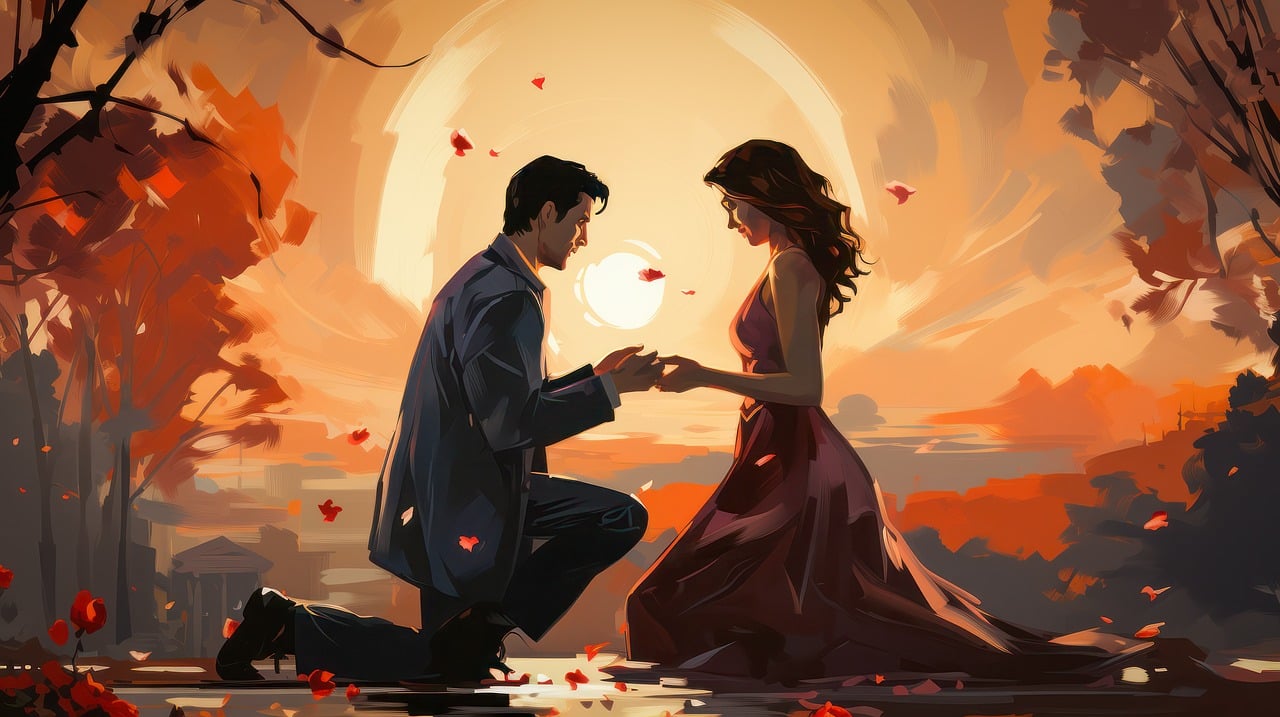
Pros of SHAP: Provides unified feature importance measure, captures interactions between features.
Cons of SHAP: Computationally expensive, interpretation complexity increases with the number of features.
SHAP values provide a unified measure of feature importance in AI models. By calculating the contribution of each feature to the model’s output, SHAP enables better understanding of model behavior.
2.5 Generative Models for Explainability
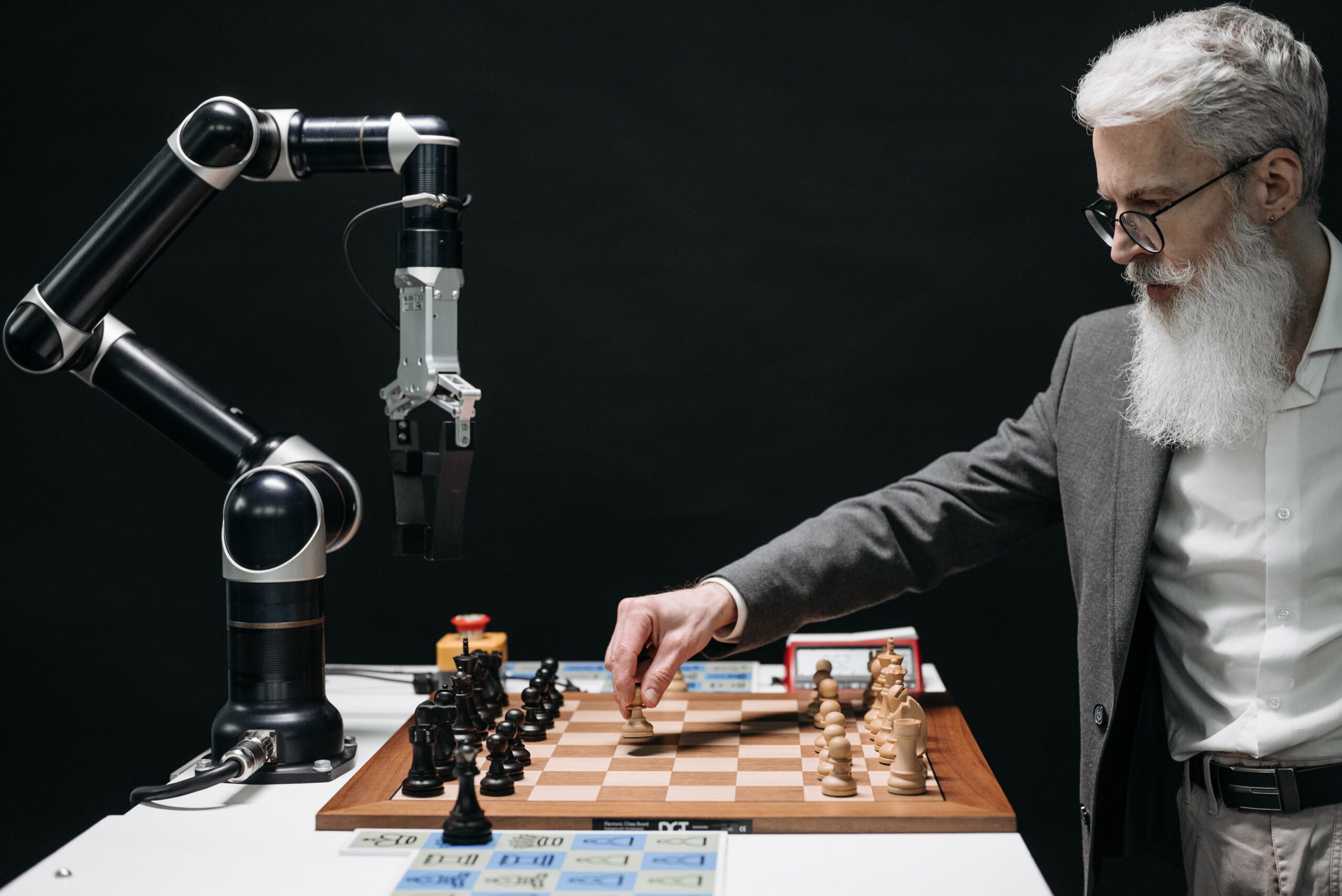
Pros of Generative Models for Explainability: Can generate counterfactual examples, offer insights into model behavior.
Cons of Generative Models for Explainability: May require large amounts of data, complex training process.
Generative models, such as Variational Autoencoders (VAEs) and Generative Adversarial Networks (GANs), can help explain AI predictions by generating counterfactual examples. These counterfactuals shed light on how changes in input affect the model’s outputs.
2.6 The Game-Changing Breakthrough – Transparent Neural Networks (TNNs)
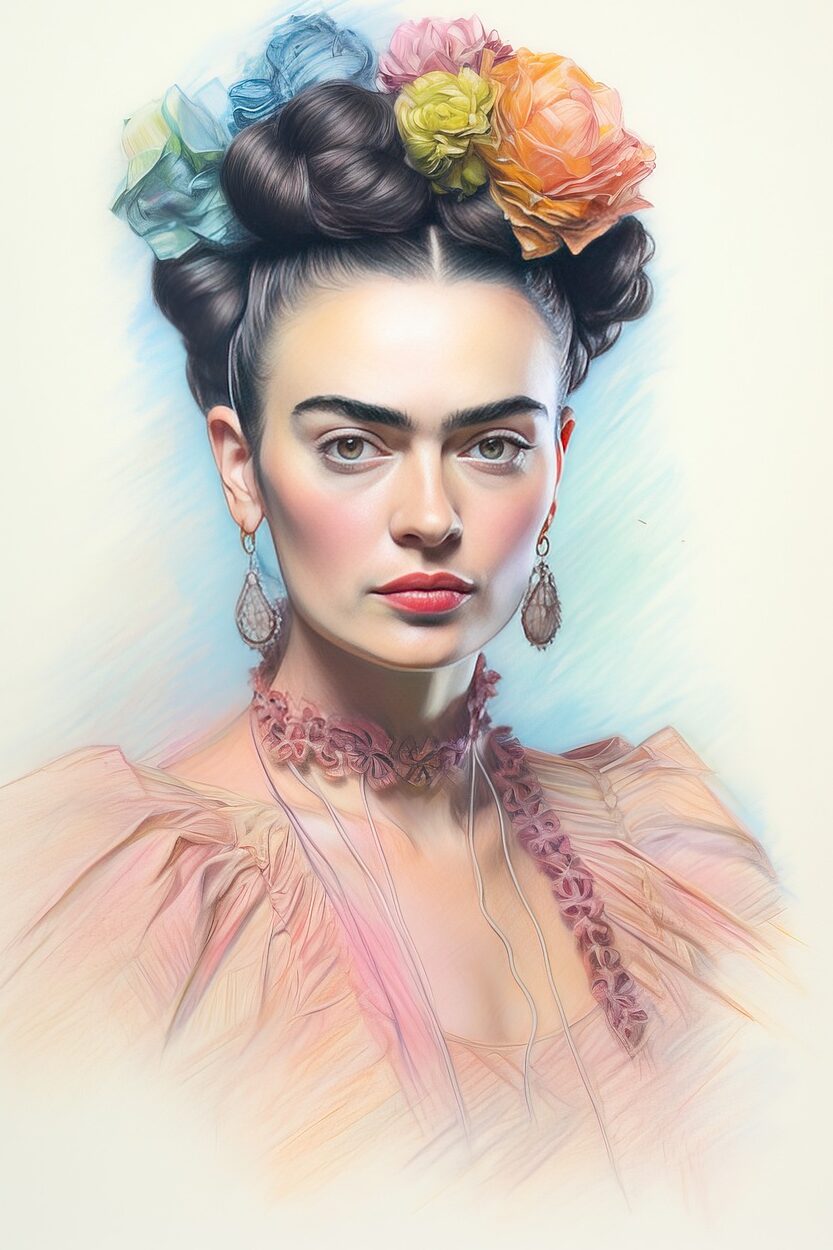
Pros of (TNNs): Explicit representations of decision-making, unparalleled explainability.
Cons of (TNNs): Potentially reduced performance compared to complex models, additional design complexity.
And now, the moment you’ve been waiting for – the AI breakthrough that will leave you astounded! Number 6 is the concept of Transparent Neural Networks (TNNs). Transparent Neural Networks are engineered to have explicit representations of their decision-making processes. TNNs use learnable rules and self-attention mechanisms to provide comprehensive and understandable explanations for their predictions, setting a new standard for AI Explainability.
3) Real-World Applications of Explainable AI
The advancements in AI Explainability are not just theoretical concepts; they have practical implications across various industries. Here are some real-world applications where transparent AI models can make a significant difference:
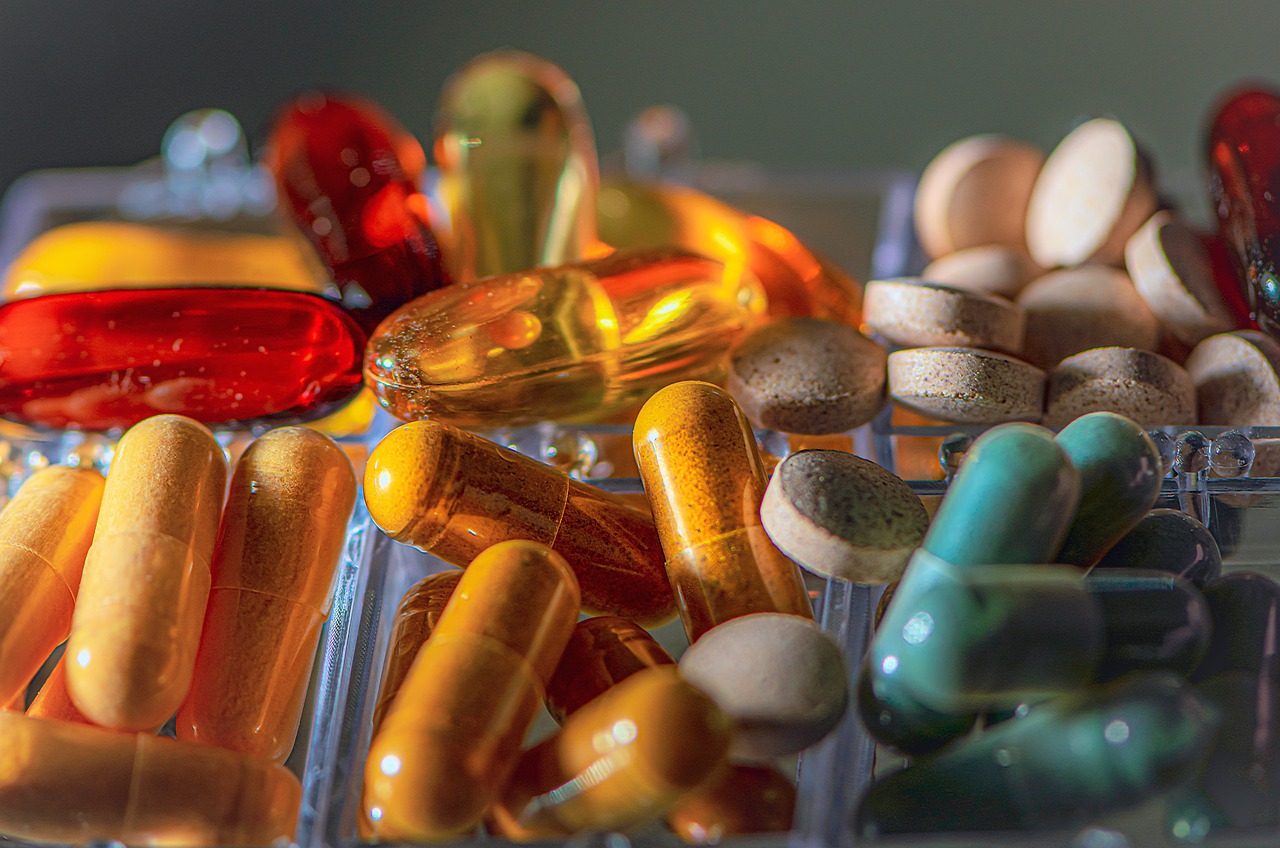
Healthcare
Transparent AI models can aid medical professionals in making critical decisions by providing clear justifications for diagnoses and treatment recommendations. The ability to understand how the AI arrived at a particular conclusion enhances trust and ensures patient safety.
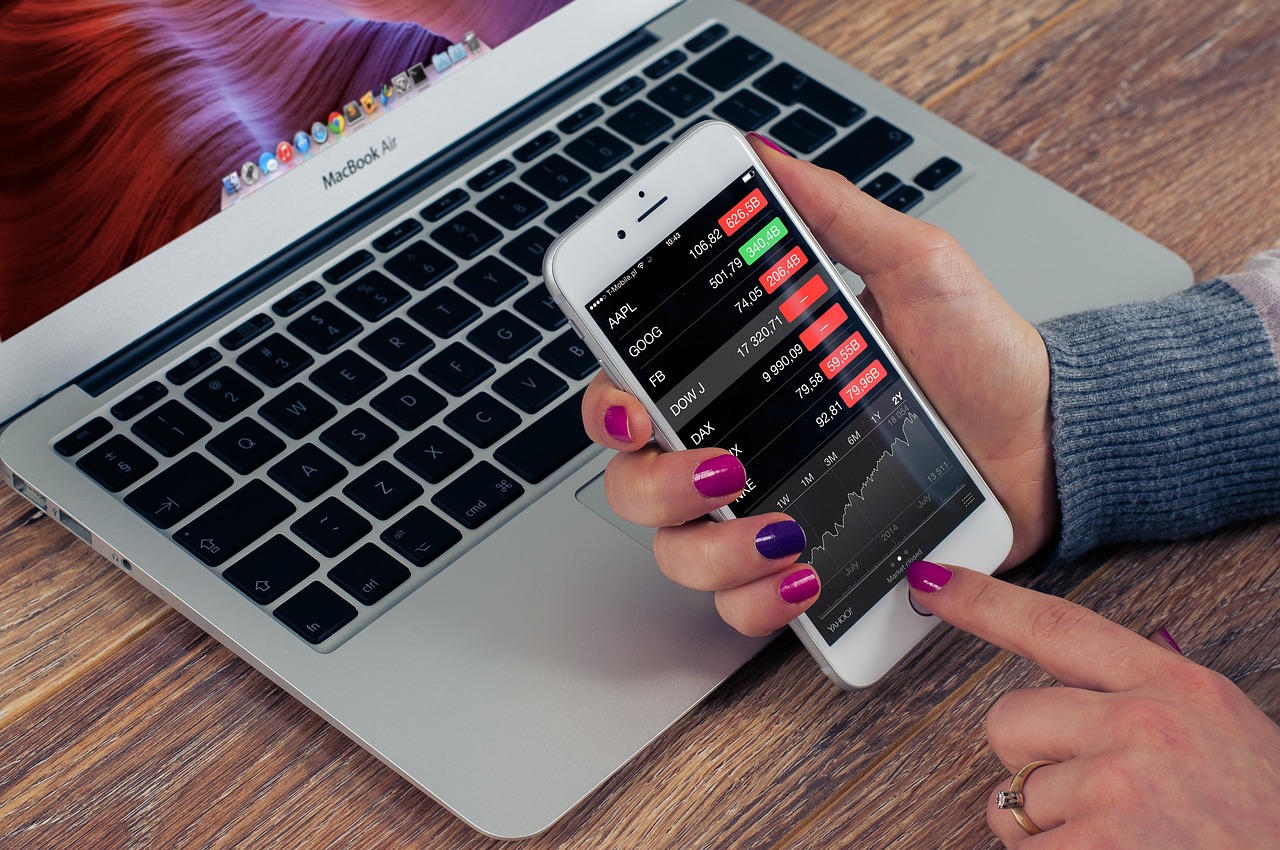
Finance
In the financial sector, explainable AI can be used for credit scoring, fraud detection, and risk assessment. Financial institutions can now have insights into the factors influencing the AI’s decisions, leading to better risk management and compliance.
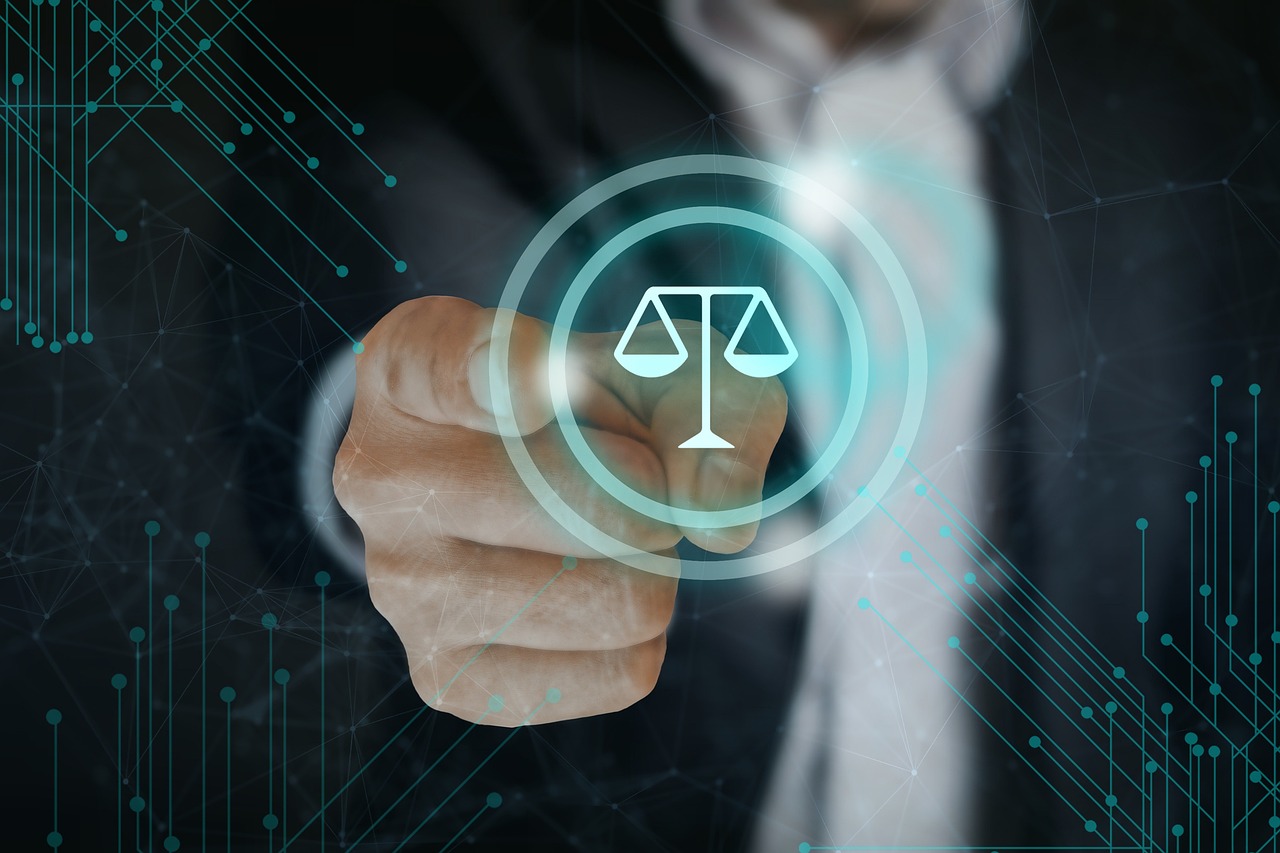
Criminal Justice
Transparent AI models can play a crucial role in the criminal justice system. Judges, lawyers, and juries can better understand the reasoning behind AI-generated recommendations for sentencing, parole, and bail decisions, ensuring fairness and reducing potential bias.
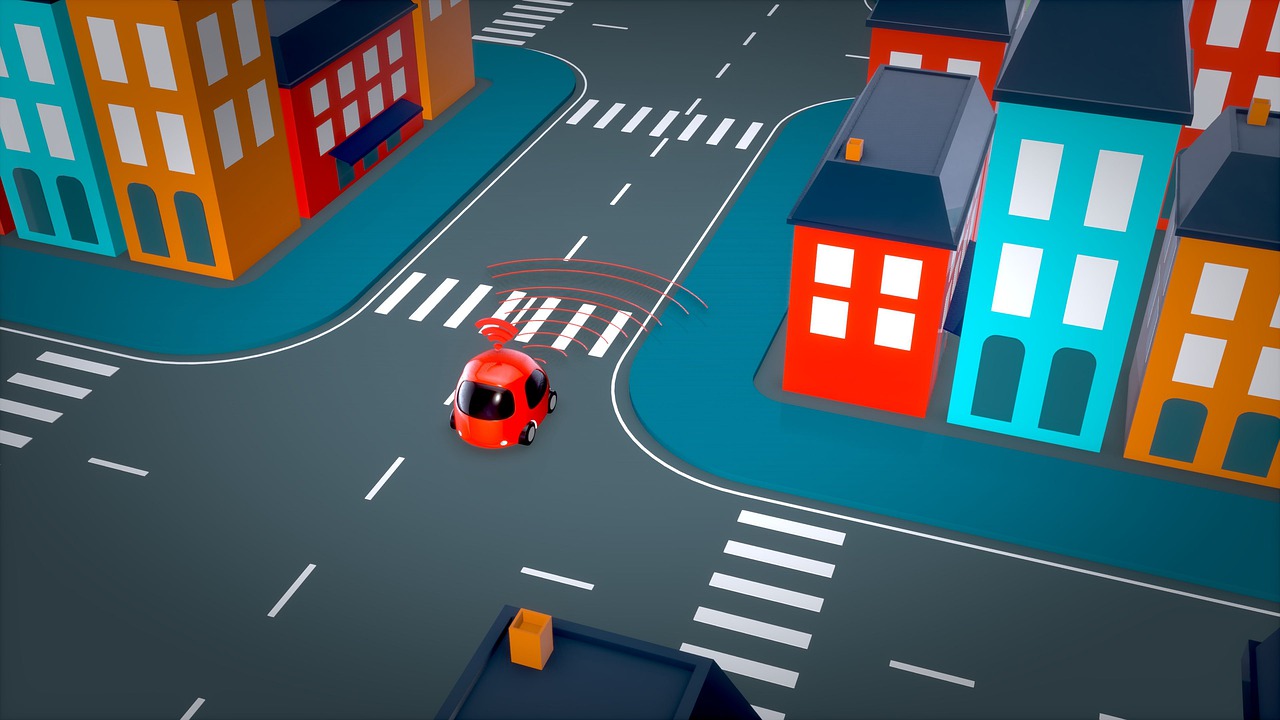
Autonomous Vehicles
Self-driving cars rely heavily on AI systems to make split-second decisions on the road. Explainable AI can provide passengers with a clear understanding of why the vehicle made certain driving choices, instilling confidence in the safety and reliability of autonomous technology.
4) Overcoming Challenges and Future Outlook
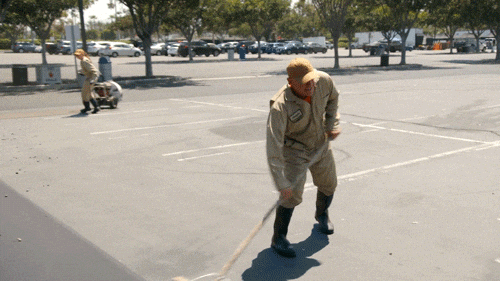
While the progress in explainable AI is promising, several challenges still need to be addressed. These challenges include balancing transparency and performance, handling complex models, and devising standardized evaluation metrics for Explainability. Researchers and industry experts continue to work together to overcome these hurdles and push the boundaries of AI transparency.
Looking ahead, the future of explainable AI holds immense potential. As the technology evolves, we can expect more sophisticated and robust approaches to interpretability. Transparent AI models are likely to become an essential requirement for deploying AI systems in critical domains, ensuring ethical, trustworthy, and accountable AI applications.
4.1 Ethical Considerations and Human-AI Interaction
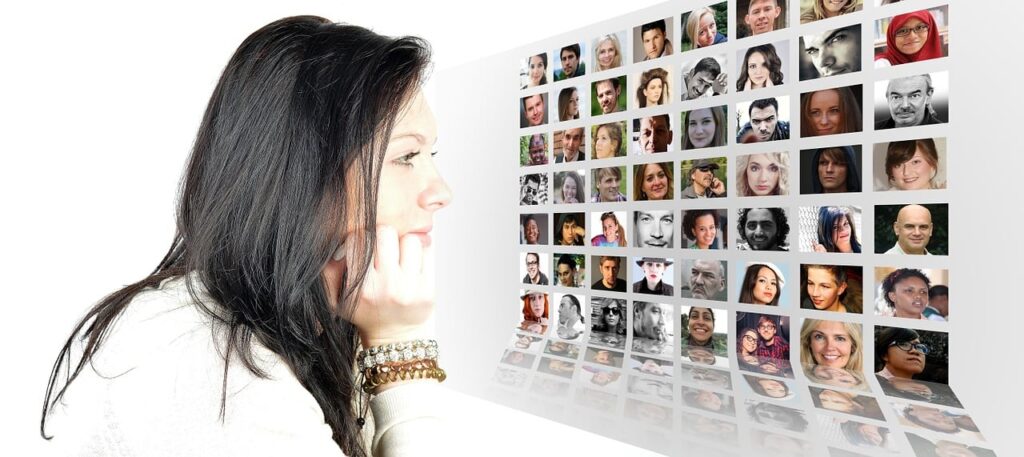
As we make AI more explainable, it is essential to address ethical considerations surrounding AI decision-making. Human-AI interaction becomes more critical as users gain access to the explanations provided by the AI systems. Ensuring that explanations are presented in a manner that is easily understandable and meaningful to humans is crucial for fostering trust and avoiding potential misinterpretations. Additionally, AI developers must remain vigilant in detecting and mitigating biases present in the data used to train AI models. Transparent AI can help expose any biases that may exist, enabling corrective measures to be taken.
4.2 Embracing the Era of Explainable AI
The era of explainable AI is upon us, and it is a transformational phase in the field of Artificial Intelligence. The Best AI Breakthroughs, including Transparent Neural Networks, are leading us towards a future where AI systems become more transparent, interpretable, and ultimately accountable for their decisions.
As individuals, developers, and organizations, it is crucial to embrace and advocate for explainable AI. By leveraging these advancements responsibly, we can harness the full potential of AI while ensuring that its applications align with ethical and societal standards.
So, are you ready to unlock the limitless potential of explainable AI? Number 6 will indeed shock you, but it represents just the beginning of an extraordinary journey into the world of AI transparency. Together, let’s shape an AI-powered future that we can all understand, trust, and benefit from!
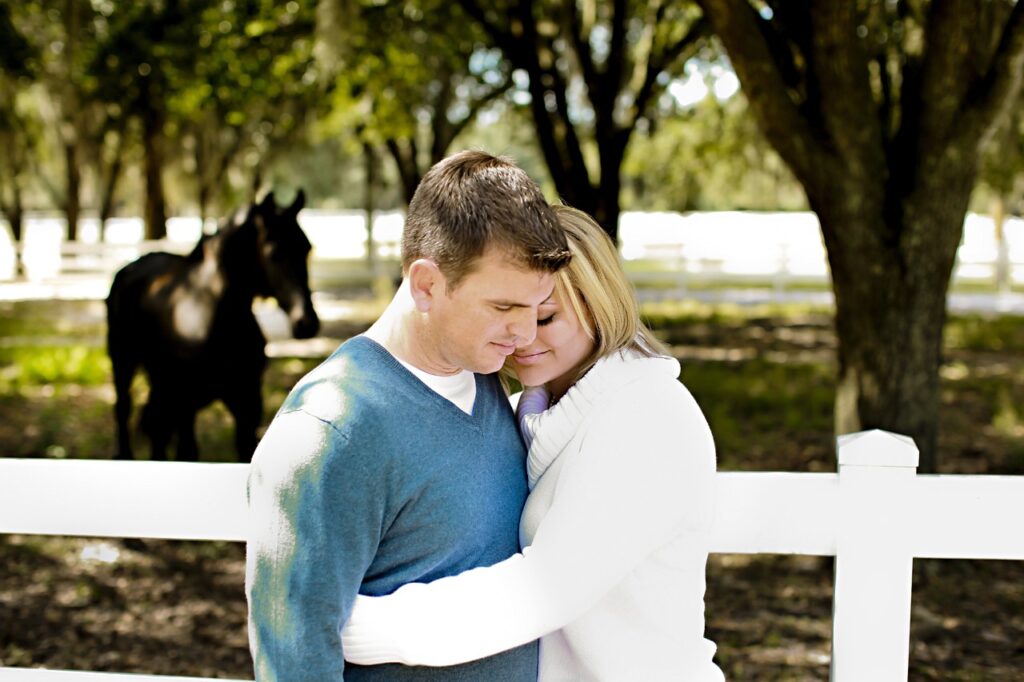
Conclusion
As we conclude this journey through the world of AI Explainability, it’s evident that significant strides have been made to overcome the limitations of black-box AI models. The Best AI Breakthroughs presented here hold the potential to revolutionize industries, foster trust in AI, and enable broader adoption of these transformative technologies. Embrace the future of explainable AI, and witness the profound impact it will have on shaping a transparent and trustworthy AI landscape!
***Remember, Number 2.6 will indeed shock you, but its implications will awe you even more! Stay tuned for more fascinating developments in the ever-evolving world of AI Explainability***
Frequently Asked Questions
Q1: What is Explainability in artificial intelligence?
A: Explainability in artificial intelligence (AI) refers to the ability of AI models and algorithms to provide understandable and transparent reasoning for their decisions and predictions. It involves uncovering the internal workings of the AI system in a way that can be easily comprehended by humans, allowing users to grasp how the model arrived at a particular output.
Q2: What are the different types of explainability?
A: There are various types of explainability techniques in AI, each serving different purposes. Some common types include:
- Rule-based explainability: AI models operate based on explicit rules, making their decision-making process easily interpretable.
- Visual explanations: Techniques like attention mechanisms and Grad-CAM provide visualizations of the features influencing the model’s predictions.
- Counterfactual explanations: Generating counterfactual examples helps understand how changes in input impact the model’s output.
- Interpretable model approximations: Techniques like LIME approximate complex models with simple interpretable models to gain insights into local predictions.
Q3: What is the concept of explainability in machine learning?
A: In machine learning, explainability is the ability of a model to clarify its decision-making process and provide understandable justifications for its predictions. The concept revolves around demystifying the black box nature of certain machine learning algorithms, enabling users to trust and validate the AI system’s outputs.
Q4: What is the difference between explainability and interpretability in deep learning?
A: While explainability and interpretability are related concepts, they have distinct meanings in the context of deep learning. Explainability refers to the AI system’s ability to provide reasons and explanations for its decisions. On the other hand, interpretability pertains to the model’s overall transparency, making it understandable and easy to interpret by humans, even without detailed explanations.
Q5: What is the explainability problem?
A: The explainability problem in AI refers to the challenge of understanding and explaining the decision-making process of complex AI models, especially those based on deep learning. As models become more sophisticated, it becomes increasingly difficult to interpret their internal mechanisms, leading to the need for innovative techniques to address this issue.
Q6: Why is explainability important in AI?
A: Explainability is crucial in AI for several reasons:
- Building Trust: Transparent models foster trust in AI systems, as users can comprehend the reasons behind their predictions.
- Accountability: Explainable AI allows developers to identify and rectify biases and errors, ensuring accountability for AI outcomes.
- Regulatory Compliance: In some domains, such as healthcare and finance, explainability is required to meet regulatory standards and ensure ethical AI practices.
Q7: What is transparency vs. explainability in AI?
A: Transparency in AI refers to making the AI system’s process and decision-making accessible to users. On the other hand, explainability goes a step further, providing not just access but clear and understandable explanations for the AI’s decisions. Transparency alone may not be sufficient if the explanations are not comprehensible.
Q8: What is explainability of algorithms?
A: Explainability of algorithms refers to the extent to which AI models and algorithms can reveal the factors influencing their decisions. It involves techniques and methods that help in understanding how the model processes input data to produce the output.
Q9: What is the explainability principle?
A: The explainability principle is a concept that suggests that AI models should provide explanations for their decisions in a way that is understandable to humans. This principle aims to enhance transparency, accountability, and trust in AI systems.
Q10: Which are two of the techniques that can be used for model explainability?
A: Two commonly used techniques for model explainability are:
LIME (Local Interpretable Model-agnostic Explanations): Approximates complex models with interpretable models for localized explanations.
SHAP (SHapley Additive exPlanations): Provides a unified measure of feature importance in AI models, helping to understand their decision process.
Q11: How does explainability contribute to trust in AI?
A: Explainability instills trust in AI systems by enabling users to understand the reasoning behind AI predictions. When users can verify the basis of AI decisions, they are more likely to rely on the system’s outputs, leading to increased trust in the technology.
Q12: What are the criteria for explainability?
A: Effective explainability in AI should meet several criteria, including:
- Comprehensibility: Explanations should be clear and understandable to the target audience.
- Accuracy: The provided explanations should accurately represent the model’s decision-making process.
- Consistency: Consistent explanations for similar inputs enhance the reliability of the AI system.
- Simplicity: Simpler explanations are easier for users to grasp and validate.
Q13: What are the three main evaluation levels of interpretability?
A: The three main evaluation levels of interpretability are:
- Algorithm level: Assessing how interpretable the individual components or algorithms within the model are.
- Model level: Evaluating the overall interpretability of the model, considering its architecture and internal workings.
- Global level: Understanding how the model behaves across different inputs and determining its generalizability.
Q14: What is explainability in AI question 3?
A: In AI, explainability refers to the AI system’s capability to provide understandable and transparent justifications for its decisions. It involves revealing the internal mechanisms of the AI model to make its decision-making process comprehensible to humans.
Q15: What does it mean for an algorithm to be interpretable?
A: An interpretable algorithm is one that allows humans to understand its internal logic and decision-making process. Users can trace how the algorithm processes input data to arrive at specific outputs, enhancing transparency and trust in the algorithm’s results.
Q16: Why is explainability important?
A: Explainability is essential for several reasons:
- Building Trust: Transparent AI models increase user trust by providing understandable explanations for their decisions.
- Detecting Bias: Explainable AI helps identify and address biases present in the data or model, promoting fairness.
- Compliance: In certain domains, like healthcare and finance, explainability is necessary to meet regulatory and ethical standards.
Q17: What are the limitations of XAI?
A: Despite its benefits, Explainable AI (XAI) has some limitations, including:
- Performance Trade-off: Some interpretable models may sacrifice performance compared to more complex, less interpretable models.
- Interpretability Complexity: As models get more complex, explaining them in a simple and meaningful way becomes challenging.
- Subjectivity: Human interpretations of explanations may vary, leading to differing opinions about model behavior.
Q18: What are the challenges of explainable AI?
A: The challenges of explainable AI include:
- Complex Models: Explaining deep learning and complex AI models is difficult due to their intricate architectures.
- Quantifying Explainability: Developing standardized metrics to measure the effectiveness of explanations is a challenge.
- Balancing Accuracy and Interpretability: Achieving a balance between model accuracy and interpretability poses a significant challenge.