To Share is to Show You Care!
Bias in AI has been a pressing issue, raising concerns about fairness and ethical considerations. In this blog post, we’ll explore actionable strategies to crush bias in AI, emphasizing emotionally intelligent approaches for a more equitable future.
1. Understanding Bias in AI
Before diving into solutions, it’s crucial to grasp the nuances of bias in artificial intelligence. Bias can manifest in various forms, such as data bias, algorithmic bias, and representation bias. Identifying these biases is the first step toward creating effective solutions.
2. The Best Strategies for Tackling AI Bias
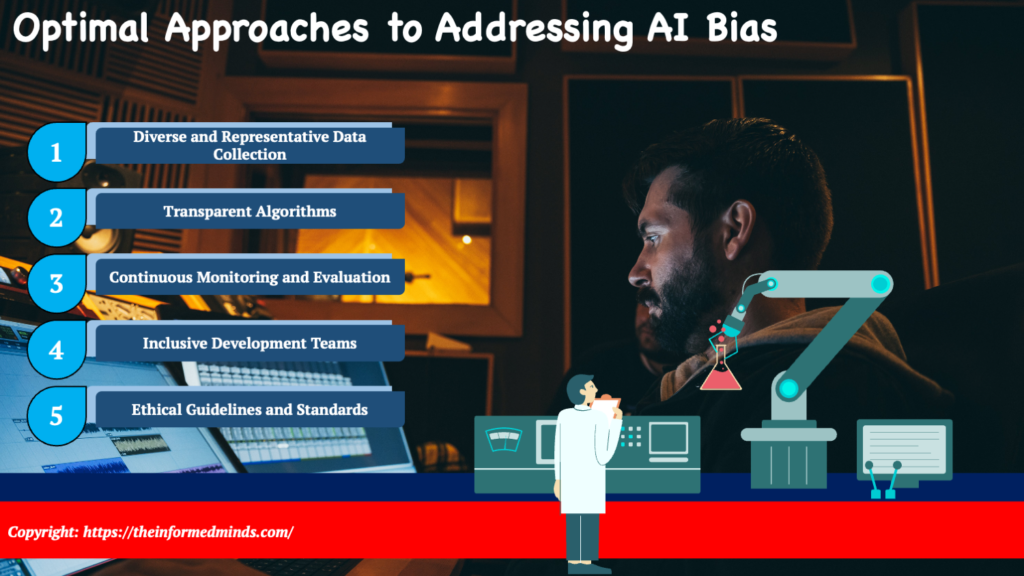
2.1 Diverse and Representative Data Collection
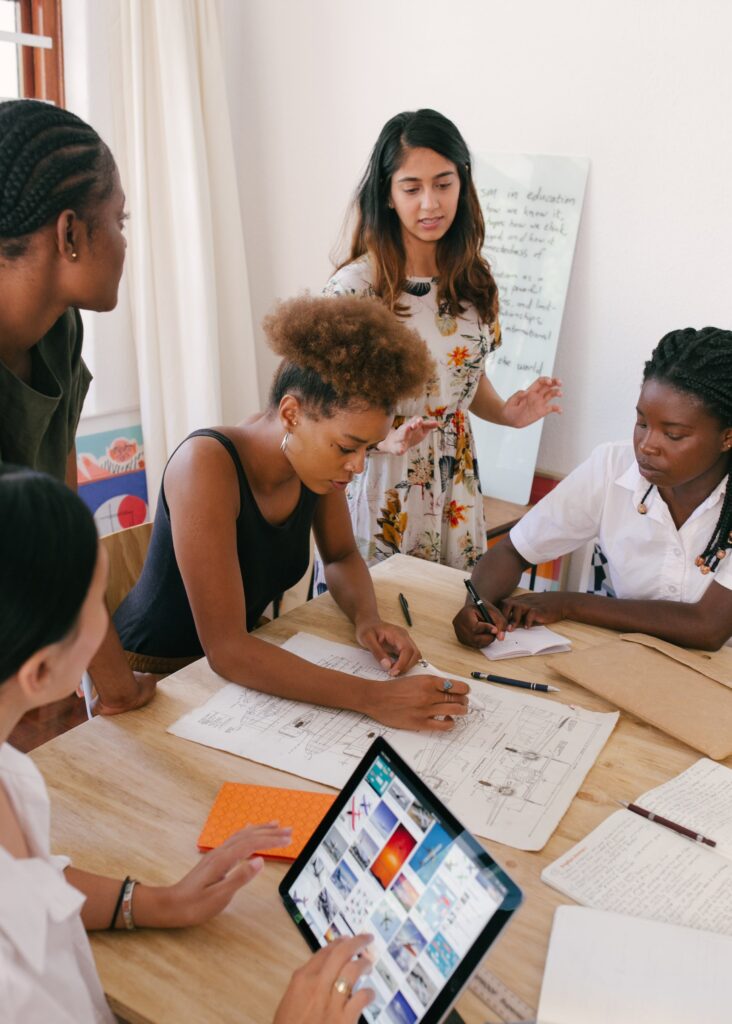
- Importance of diverse datasets: Ensure that the data used to train AI models is diverse and representative of the real-world population. This helps in preventing bias by exposing the model to a wide range of examples and scenarios.
- Strategies for ensuring representation in training data: Implement techniques such as oversampling underrepresented groups, using stratified sampling, or incorporating data augmentation to ensure fair representation across different demographics.
- Tools for identifying and mitigating data bias: Utilize tools like fairness indicators and bias detection algorithms to identify and address bias in training data. Regularly audit datasets to ensure ongoing fairness.
2.2 Transparent Algorithms
- The role of transparency in addressing algorithmic bias: Transparency in algorithms allows developers and users to understand how decisions are made. Implementing transparency helps in identifying and rectifying biased patterns in the decision-making process.
- Implementing explainable AI for better understanding: Develop algorithms that provide clear explanations for their decisions. Explainable AI techniques, such as model-agnostic interpretability methods, can help users understand the reasoning behind AI-generated outcomes.
- Tools and frameworks for algorithmic transparency: Leverage tools like LIME (Local Interpretable Model-agnostic Explanations) or SHAP (SHapley Additive exPlanations) to enhance the transparency of machine learning models.
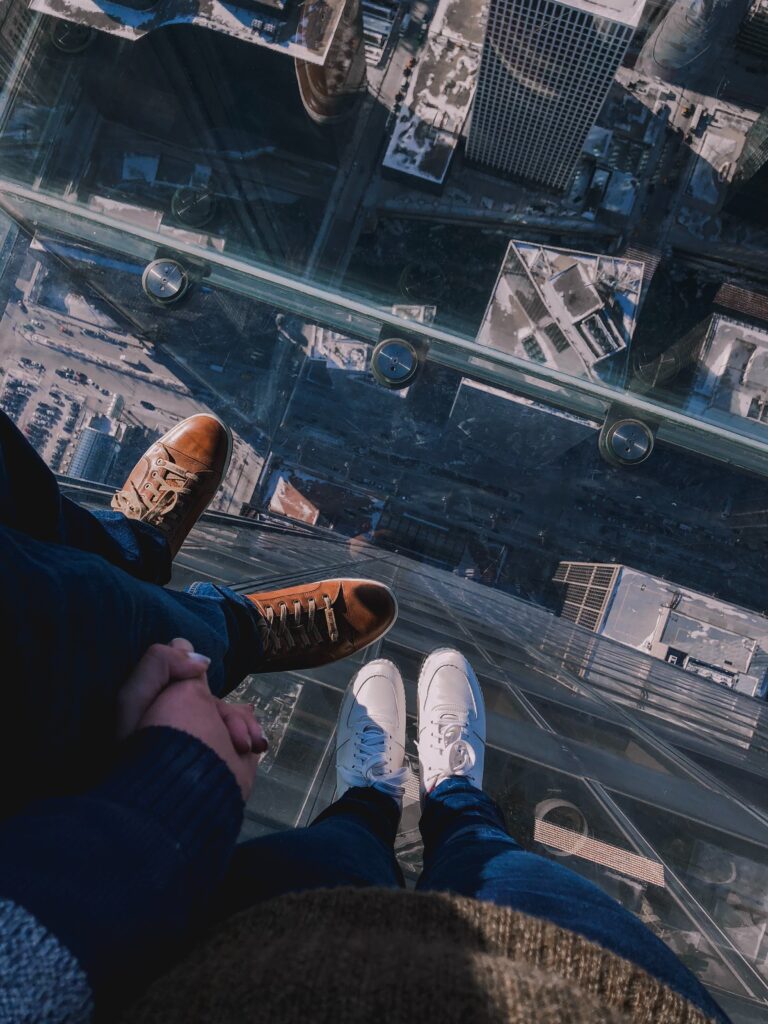
2.3 Continuous Monitoring and Evaluation
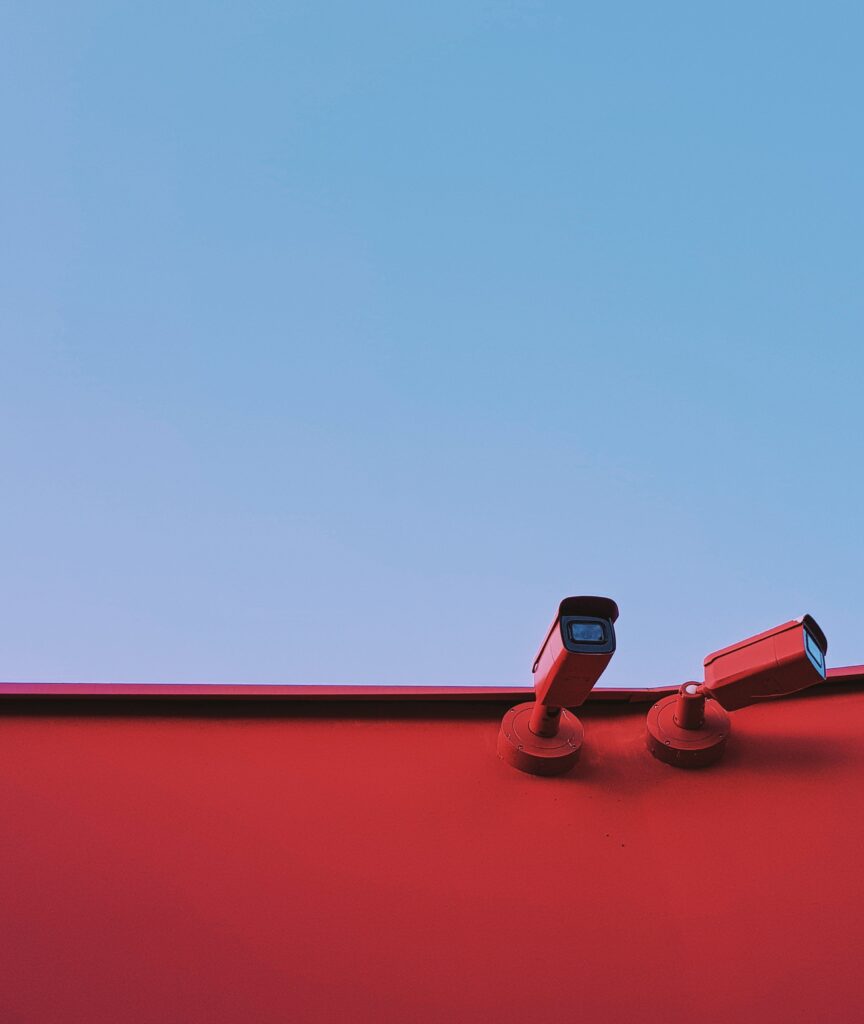
- The need for ongoing assessment of AI systems: Implement continuous monitoring of AI systems to detect and address bias over time. Regular evaluations ensure that the model’s performance remains fair and unbiased throughout its lifecycle.
- Establishing feedback loops for continuous improvement: Create mechanisms for collecting feedback from users and stakeholders. Establishing feedback loops helps in identifying and rectifying bias based on real-world experiences and concerns.
- Key performance indicators for evaluating bias reduction: Define key performance indicators (KPIs) to measure the success of bias reduction efforts. KPIs may include fairness metrics, accuracy across different demographic groups, and user satisfaction.
2.4 Inclusive Development Teams
- Building diverse teams for AI development: Foster diversity within AI development teams by including individuals from different backgrounds, experiences, and perspectives. Diverse teams are more likely to recognize and address bias during the development process.
- Fostering an inclusive culture in tech: Create an inclusive environment where all team members feel comfortable expressing their opinions and concerns. Inclusive cultures promote open discussions about potential biases and encourage collaboration in finding solutions.
- The impact of team diversity on mitigating bias: Diverse teams bring a range of perspectives that can help identify and rectify biases that may not be apparent to a homogenous group. This leads to more robust and unbiased AI systems.
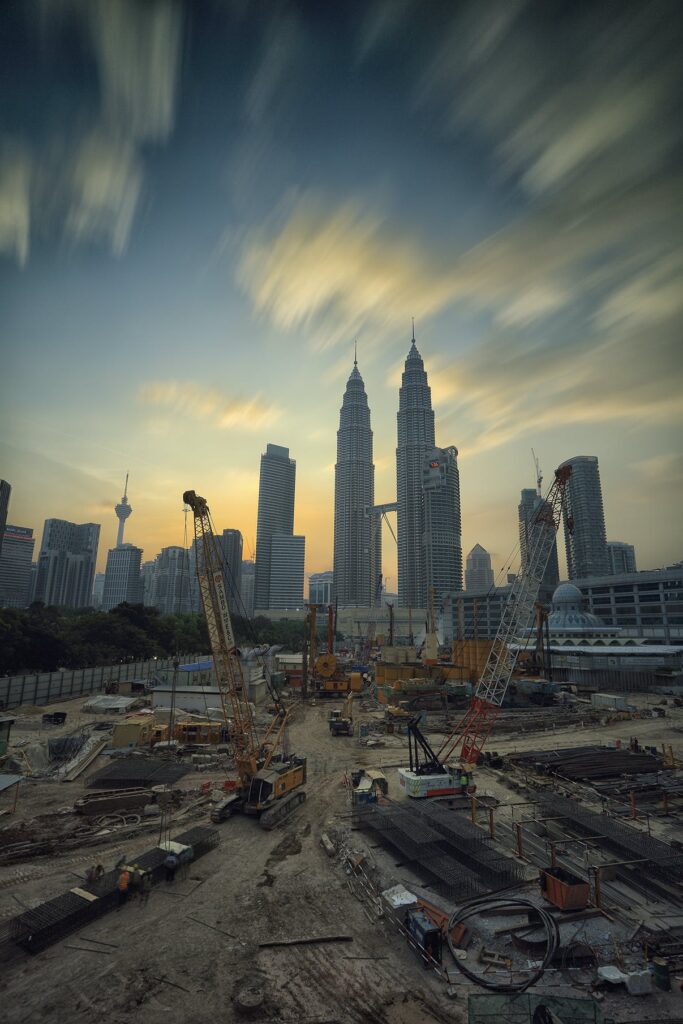
2.5 Ethical Guidelines and Standards
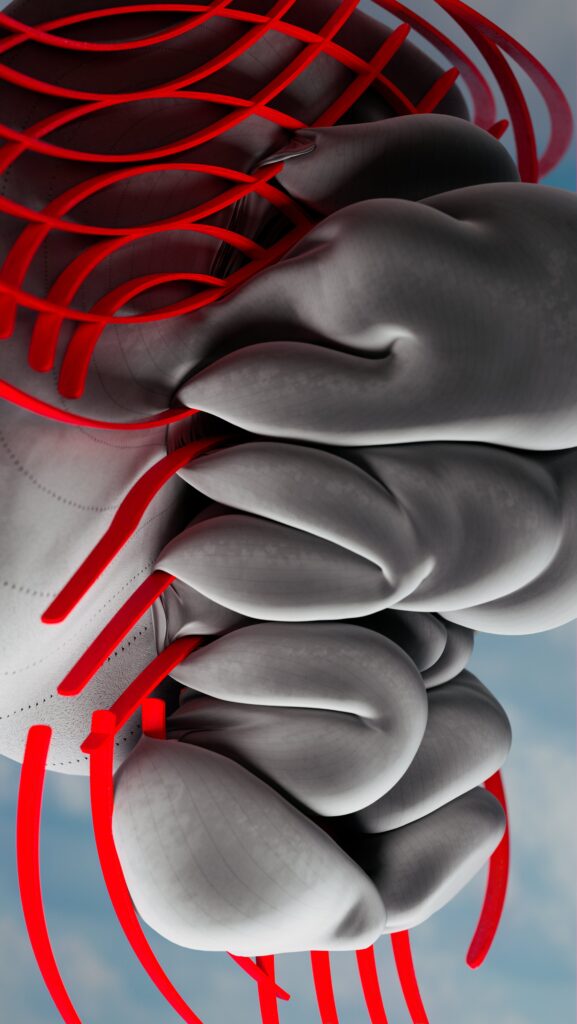
- Incorporating ethical considerations in AI development: Integrate ethical considerations into the development process. Consider the potential societal impact of AI systems and adhere to ethical guidelines to ensure fairness, transparency, and accountability.
- Existing standards and guidelines for ethical AI: Adhere to established ethical AI standards and guidelines, such as those provided by organizations like the IEEE or initiatives like the Partnership on AI. These frameworks offer principles and best practices for developing ethical AI.
- The role of regulatory frameworks in shaping responsible AI practices: Stay informed about and comply with regulatory frameworks that address AI ethics. Some regions may have specific guidelines and regulations governing the development and deployment of AI systems.
3. Emotionally Intelligent Approaches
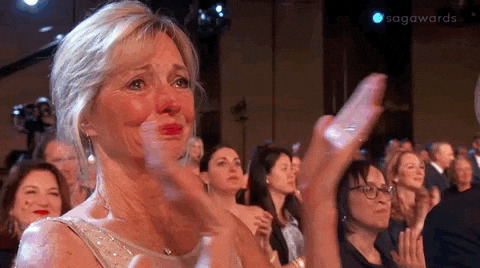
3.1 Empathy in Algorithm Design
- Designing algorithms that consider diverse perspectives: Infuse empathy into the algorithm design process by considering the diverse perspectives and experiences of the user base. This involves understanding how different groups may be affected by AI decisions.
- Incorporating user feedback to understand emotional impact: Actively seek and incorporate user feedback to understand the emotional impact of AI-generated outcomes. This feedback loop can help developers identify and address emotionally charged issues.
3.2 Stakeholder Engagement
- Engaging with diverse stakeholders to gather insights: Engage with a wide range of stakeholders, including end-users, advocacy groups, and domain experts, to gather diverse insights. Understanding the perspectives of different stakeholders helps in designing fair and unbiased AI systems.
- Understanding the emotional concerns of different user groups: Recognize and address the emotional concerns of various user groups. This involves conducting empathy-driven interviews, surveys, and usability studies to uncover emotional responses to AI interactions.
3.3 Education and Awareness
- Raising awareness about bias in AI and its consequences: Educate both developers and users about the existence and consequences of bias in AI systems. Increased awareness promotes a collective commitment to addressing bias and encourages responsible AI practices.
- Educating users and developers on ethical AI practices: Provide resources and training to users and developers on ethical AI practices. This includes understanding the implications of biased AI and how to actively contribute to mitigating bias in AI systems.
Conclusion
Crushing bias in AI requires a multi-faceted approach, combining technical solutions with emotionally intelligent strategies. By implementing diverse and representative datasets, transparent algorithms, continuous monitoring, inclusive teams, and ethical guidelines, we can pave the way for a more equitable and emotionally intelligent AI future.
Frequently Asked Questions
Q1: Why biased outcomes in AI are difficult to identify?
Answer: Biased outcomes in AI are challenging to identify because biases can be subtle, complex, and deeply embedded in the data and algorithms. Moreover, the vast and diverse datasets used for training AI models may contain implicit biases that are not immediately apparent during the development and testing phases.
Q2: What are bias problems with AI?
Answer: Bias problems in AI refer to situations where AI systems exhibit unfair or discriminatory behavior, often reflecting the biases present in the data used for training. These issues can lead to unequal treatment of different groups, reinforcing societal prejudices and potentially causing harm.
Q3: How to detect bias in AI?
Answer: Detecting bias in AI involves using specialized tools and techniques, such as fairness indicators, bias detection algorithms, and fairness metrics. Additionally, thorough analysis of model outcomes across different demographic groups and continuous monitoring during the development lifecycle can help identify and address bias.
Q4: What are the limitations of AI bias?
Answer: Limitations of AI bias include the challenge of completely eliminating bias, the difficulty in foreseeing all potential biases during development, and the risk of perpetuating existing societal biases. Additionally, biases may arise from unforeseen interactions between variables in complex datasets.
Q5: Why is algorithmic bias hard to diagnose?
Answer: Algorithmic bias is difficult to diagnose because biases can be unintentional, influenced by complex interactions in data, and not always evident in the outcomes. Lack of transparency in some algorithms and the dynamic nature of data make it challenging to identify and diagnose bias effectively.
Q6: How can bias in AI be corrected?
Answer: Correcting bias in AI involves a multi-faceted approach, including using diverse and representative datasets, transparent algorithms, continuous monitoring, and implementing ethical guidelines. Additionally, fostering an inclusive development environment and incorporating user feedback contribute to the ongoing correction of bias.
Q7: What are the 2 main types of AI bias?
Answer: The two main types of AI bias are data bias, arising from skewed or unrepresentative datasets, and algorithmic bias, which occurs when the design or implementation of the algorithm itself introduces unfairness or discrimination.
Q8: What is the root cause of AI bias?
Answer: The root cause of AI bias often lies in the data used to train models. If the training data reflects societal biases or is not diverse and representative, the AI system may learn and perpetuate these biases in its decision-making processes.
Q9: What is one potential cause of bias in an AI system?
Answer: One potential cause of bias in an AI system is a biased training dataset. If the data used to train the model contains imbalances or reflects societal prejudices, the AI system can inadvertently learn and replicate these biases in its predictions.
Q10: What is AI bias in simple words?
Answer: AI bias, in simple terms, refers to the presence of unfair or discriminatory outcomes in artificial intelligence systems. It occurs when the AI system produces results that reflect and perpetuate existing biases present in the data used for training.
Q11: What are the three sources of biases in AI?
Answer: The three sources of biases in AI are data bias (biased training data), algorithmic bias (inherent biases in the design or implementation of algorithms), and user interaction bias (biases introduced through user feedback or interaction patterns).
Q12: What is an example of bias in AI systems?
Answer: An example of bias in AI systems is a facial recognition algorithm that performs more accurately for lighter-skinned individuals than for darker-skinned individuals, reflecting the biases present in the training data used to develop the algorithm.
Q13: Can we eliminate bias in AI?
Answer: Completely eliminating bias in AI is challenging, but ongoing efforts focus on minimizing and mitigating biases through diverse datasets, transparent algorithms, and ethical development practices. Continuous monitoring and addressing biases as they emerge contribute to improvement.
Q14: Can artificial intelligence have bias?
Answer: Yes, artificial intelligence can have bias. Bias can be unintentionally introduced during the development process, particularly through biased training data and algorithmic design. Addressing and minimizing bias is a critical aspect of responsible AI development.
Q15: What is bias in AI 2023?
Answer: As of my last knowledge update in January 2023, I don’t have specific information about bias in AI in 2023. It’s recommended to stay updated on the latest developments and research in the field to understand any advancements or changes in addressing bias in AI systems.
The Informed Minds
I'm Vijay Kumar, a consultant with 20+ years of experience specializing in Home, Lifestyle, and Technology. From DIY and Home Improvement to Interior Design and Personal Finance, I've worked with diverse clients, offering tailored solutions to their needs. Through this blog, I share my expertise, providing valuable insights and practical advice for free. Together, let's make our homes better and embrace the latest in lifestyle and technology for a brighter future.